Artificial Intelligence(AI) in Railways: A Technical Deep Dive into the Future of Transport
8/29/20232 min read
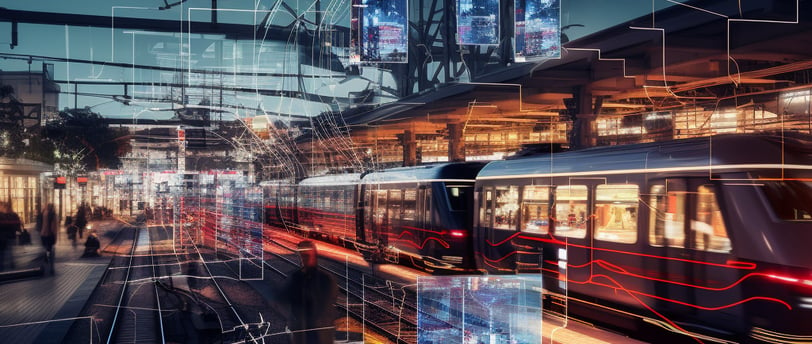
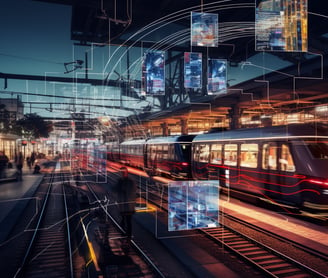
Artificial Intelligence in Railways: A Technical Deep Dive into the Future of Transport
The intersection of Artificial Intelligence (AI) and railway operations isn't just a contemporary innovation; it's a pioneering step toward redefining transportation's very core. With an intricate mesh of algorithms, sensors, and data analytics, AI offers transformative solutions to longstanding railway challenges. Here's a technical exploration into how AI is revolutionizing railways.
1. Predictive Maintenance with Machine Learning
Data Collection: High-frequency sensors collect myriad data points from tracks, engines, carriages, and signaling systems. These include vibrations, temperatures, and acoustic signals.
Algorithmic Analysis: Machine Learning (ML) algorithms, particularly regression models and neural networks, are trained using this data. Over time, these models become adept at recognizing patterns indicative of potential equipment failures.
Implementation: Predictive analytics tools, when integrated into the railway's maintenance management system, can schedule proactive repairs, thus reducing unplanned downtimes.
2. Enhancing Safety through Computer Vision
Detection Systems: Advanced computer vision systems process thousands of hours of footage from track-side cameras. They're designed to detect irregularities ranging from track obstructions to wear and tear.
Response Protocols: Once an anomaly is detected, the system can instantly alert operators or even integrate with automated control systems to slow down or halt trains if a significant risk is identified.
3. Optimizing Traffic with Reinforcement Learning
Dynamic Scheduling: By employing reinforcement learning, traffic management systems can learn optimal train scheduling by continually adjusting to variables like passenger flow, weather conditions, and technical disruptions.
Real-time Adjustments: Advanced AI models, built on recurrent neural networks (RNNs), can predict sudden changes in demand or capacity, allowing for immediate rescheduling.
4. Personalized Passenger Experiences via Natural Language Processing (NLP)
Chatbots & Virtual Assistants: Using NLP, railways can offer AI-driven assistants to passengers, capable of understanding and responding to complex queries related to schedules, fares, and services.
Sentiment Analysis: Feedback from passengers can be processed using sentiment analysis, helping operators gauge satisfaction levels and make necessary improvements.
5. Cybersecurity fortified with Deep Learning
Intrusion Detection: Deep learning models, especially convolutional neural networks (CNNs), are deployed to monitor data traffic, identifying potential cyber threats based on known attack patterns.
Data Integrity: AI algorithms ensure data consistency across distributed ledgers, mitigating risks associated with data tampering.
6. AI-Driven Infrastructure Planning
Predictive Analytics: By processing vast datasets that include urban growth patterns, demographic shifts, and environmental data, AI tools can provide insights for future railway expansion or modification plans.
Simulation: AI-driven simulations can model future scenarios, helping planners make informed decisions on infrastructure investments.
Conclusion: Merging Technical Prowess with Operational Brilliance
The blend of AI's technical sophistication with railway operations promises a paradigm shift. With each algorithm and sensor, we move closer to a future where railways aren't just faster and more efficient but also remarkably intelligent.